How Standards Drive Innovation
Download MP3Hi everyone!
Welcome to Hard Problems, Smart
Solutions, the Newfire Podcast.
I'm Will Crawford, Head of
Advisory Services and CTO at
Newfire Global Partners, and I'll
be your host for this episode.
Today I'm talking with Dr. Paulo Pinho.
Over his career, Paulo has straddled
the line between care delivery,
payer technology and entrepreneurship
including stints as Chief Medical
Officer of Discern Health, and his
VP and Medical Director of Innovation
for Diameter Health and Availity.
He's also a practicing physician.
Today we're talking about the
evolution of healthcare standards
and the importance of clinical
informatics for healthcare innovation.
We'll explore how advances in
standards have enabled new kinds of
applications, including ones that
leverage AI and some of the challenges
that come with those changes.
We're also going to talk about
the transition from clinical
medicine to the corporate world.
And how as a non-clinician
you can work more effectively
with your clinical partners.
Dr. Pinho, welcome to the podcast.
Thanks, Will.
I appreciate being here and, uh, looking
forward to, to digging in for sure.
So before we dive into, uh, specific
topics, could you just tell us a little
bit about your journey in healthcare?
Uh,
Yeah, so a, a little bit
of an unorthodox journey.
I, um, you know, started out
in, um, in clinical practice
right outta medical school.
I'm a primary care, uh,
physician, board certified in
internal medicine and pediatrics.
Um, and worked in both disciplines,
had my own practice that I was able
to build up and, you know, started to
experience many of the challenges that
exist in healthcare delivery, um, from
a, a data standpoint, from a quality
of care standpoint, the defining what
quality care was for individuals.
Ended up selling my practice to a hospital
system and, uh, it was early on in the
consolidation of hospital practices and
eventually I, um, uh, actually made the
move to more executive health roles,
uh, initially with, uh, Prudential,
where a lot of my work focused on things
like thoughtful benefits, benefits
design, um, really creating sort of
an engaged and empowered workforce,
you know, using, uh, benefits design
as the foundation for that and, you
know, really using it to drive employee
engagement, et cetera, and then moved out
into the healthcare data standards world,
predictive analytics world and AI world.
So it took me a while to kind of realize
the scale and the opportunities that
exist, uh, when you use technology to
enable the way healthcare is delivered.
And I think that now more than ever,
it's, you know, becoming a need.
I, I think you have to mitigate some
of that supply demand mismatch with
thoughtful, uh, technology solutions.
And so I'm excited to be part of this, you
know, new wave of tech-enabled healthcare.
So for people listening who you know,
don't come from that clinical informatics
background, uh, could you just talk a
little bit about why data standardization
for these applications is so complicated?
Yeah, so I think dating back to the
meaningful use days, it's uh, it's
really been a significant challenge.
Data in healthcare by definition
is multi-source and multi-format.
It's been increase, there's an
increasing amount of medical data
that's created for each individual with
nearly a thousand percent increase in,
in data capture on individuals over
the course of the last 10, 10 years.
Uh, and we also know that medicine
is becoming increasingly complex
to the point that the, this full
body of medical knowledge is,
uh, increasing at rapid paces.
I mean, we know that, uh, medical
knowledge doubling, you know, as of
2020 was at 73 days, and so it's only
shorter at this stage of the game.
We know that there has been sort of
a challenge in healthcare in terms of
evolving off of, uh, legacy systems.
Uh, we know that despite the fact that
meaningful use was, uh, intended to drive
data interoperability, it really created
a bunch of different formats of data could
be captured within provider's offices.
There were, uh, different
electronic health record platforms.
Each had their different way
of sort of collecting data.
Uh, each had their different key pieces
of information and sort of, and, and
its storage in different areas of
the electronic health record chart.
And then, you know, you could have
two separate implementations of the
same electronic health record in
two different hospitals that were
across the street from one another.
And the way they captured
data was very, very different.
So, um, despite the fact that
meaningful use intended to sort of
wrangle some of this in, it really
created a bunch of different languages.
And then on top of that, a bunch
of different dialects as well.
Um, and we know that the language
of clinical data capture was very
different when you're capturing data
for clinical intent versus when you're
capturing data for billing purposes.
Uh, we know that providers, some of
the data that we, we capture when we
see patients tends to be, you know,
very structured codified data that
we pulled out from pull down menus.
Um, but in other times you really
can't tell a full narrative story,
um, unless you actually type in pros.
And so some of that's made data
standardization really, uh, complex.
I think on top of that, we're
starting to see data capture in a
bunch of different formats that we
never thought would be, uh, data,
you know, methods of data capture.
Uh, we know that data is captured,
you know, outside of electronic health
records that's really meaningful to
healthcare delivery, for example.
There's an increasing the amount
of wearables that that, that are
out there, and we're seeing people
diagnosed with atrial fibrillation
for the first time, you know, from
their Apple watch, or with sleep wake
disturbances from a wearable ring.
We know that there's social data aspects,
uh, that that, that, you know, can't
necessarily be capturing the same way
from electronic health records.
And so ultimately, you know, the types of
data that really have become meaningful
to today's healthcare delivery in a,
in a personalized fashion, um, have
become too difficult to consolidate.
There is a challenge in sort of
the volume, velocity, variety of
clinical data and nonclinical data
that drive healthcare outcomes.
Um, and, and that's I think probably
where the biggest challenges lie.
And there is, there are increasing
challenges when it comes to, uh, data
privacy, data security, um, et cetera.
And so, you know, while there's a lot
of opportunity with the, the sheer
amounts of data that we're collecting
in healthcare, I think the challenges
and the complexities of the different
ways that data is, is, is collected,
the different languages and dialects have
really made it so that interoperability,
despite its most pure intent, has have,
has really been elusive for the healthcare
industry at the stage of the game.
So the mechanics are there, but the
sort of semantic agreement on what
gets exchanged over those pipes,
there's still a lot of work to do.
When you talk about medical knowledge
doubling every 73 days, just tell us
a little bit more about what knowledge
that is.
We see this right now.
I mean, I, I remember practicing
medicine while I was in residency.
I would say that, when I first
started in residency, HIV, for
example, was an inpatient disease.
We managed, I would say that probably 70,
80% of the patients that I was taking care
of on the wards, um, even in the pediatric
wards were HIV positive individuals
that had an opportunistic infection.
By the time I graduated residency, HIV had
shifted to largely in outpatient disease.
Um, you know, focused on giving
people the right highly-active
anti-retroviral therapy, um, to prevent
some of these opportunistic illnesses.
And so, um, we didn't
have inpatient disease.
It was a complete shift.
I mean, I would say that if I
had 20% or less of my patients
having, you know, HIV, um, that was
probably a, a large representation
by the time presidency was over.
We're seeing this with cancer care.
You know, when you look at how cancer has
has been treated up until now, it's really
been this sort of race against time.
You know, we're providing the body with
a poison in the hopes that killing the
cells that are responsible for this
tumor happens at a rate of speed that's
quicker than killing the cells that you
know are keeping a human being alive.
Um, and you know, now with more targeted
therapies that are, you know, molecular
based and focused on cell surface
receptors and really personalized to
the type of cancer that someone has,
you know, we're really able to sort of
augment not only the quantity of life for
individuals, um, who have these cancers,
but also the quality of life because
that side effect burden just isn't there.
And so, you know, I think every era
is defined by a clinical illness
or a set of clinical illnesses.
I would say that my residency training
was defined by sort of that rapid
evolution in, in, in HIV treatment.
And I think future generations is, are
gonna be the cancer personalization
and the cardiovascular disease
personalization that exists.
And these things are
happening very rapidly.
I mean, when we look at cancer care,
you know, I think that we're gonna have
a generation of clinicians, 10, 15, 20
years from now, they're gonna look back
and say, wait, let me get this straight.
This is how you used to treat cancer?
You used to give people poison
and hope that it wouldn't kill
them and kill the, kill the tumor.
This is the kind of, you know, information
that's doubling and doing so very rapidly.
It's not limited to cancer or HIV.
We see it in cardiovascular disease
and, you know, we see the ability
to personalize medicine, to do
it in minimally invasive ways.
It's changing the access opportunities
and the quality of care that we deliver,
um, in ways that we never thought
would be possible 10, 15, 20 years ago.
So I, I think that's a great example.
I mean, I, I have some people in my
life who have benefited from some of
those targeted cancer therapies, and
it's, it's really been remarkable and
it's been very grateful for that because
it would've been a very different
story, even a, even a decade ago.
But bringing those two themes together,
I, there's a lot more information around
the patient and then there's a lot more
knowledge to apply that information to.
So how are providers keeping
their head above water?
Yeah, I think, um, medical education has
definitely needs to, to pivot and I think
it already has started pivoting, obviously
providing sort of an evidence-based
strategy for literature review.
For how, and we saw this during the
COVID Pandemic, there were tons of
articles that were released and some of
them were sort of in holding patterns,
and we needed that data as clinicians
to be able to make informed decisions
about our patients and how do we
do that in a way that's thoughtful?
How do we do it to make sure that
what we are releasing to clinicians
who are using these to kind of make
decisions about their patients is done
so in an informed and an appropriate
way and that it's not quackery.
And so I think that part of our medical
education system now has evolved to
really be very critical of medical
literature and sort of some of the
technology adoption that's happened.
Um, and I think that was really
ushered in by the COVID Pandemic.
Um, it's been ushered in by the
need to personalize, you know,
healthcare for individuals.
Physicians, nurses, nurse practitioners,
physician assistants, they all recognize
at the stage of the game that, you know,
having that information accessible in
the quickest possible way is important.
So you see people using
smartphones on a regular basis,
or tablets on a regular basis.
You see electronic health records
embedded with solutions that allow
providers to look for critical studies
that may help sort of guide care.
You see clinical decision support systems
that are being embedded in electronic
health record workflows that are guiding
clinicians to make the right decision
and really sort of absolving them of
some of the administrative hurdles
of the daily practice of medicine.
And I think you see the advent of
solutions that are coming up that
you know, obviously need to be done.
So with a lot of responsible use
of comfort level with artificial
intelligence, comfort level with things
like large language models, comfort level
with things like ambient listening and how
that factors into the way data is captured
from a patient at the point of care.
And so I think there's whereas medicine
training tended to be very apprenticeship
based in my, in my days of training,
I think there's a lot more flexibility
built into the way residents are trained
in today's day and age to not only sort
of retain some of that apprenticeship
training, but also give people the
flexibility to go out and sort of
experiment with new technologies of data
capture, new technology, of, of grabbing
insights about diseases and treatments.
And so I'm, I'm excited at the
evolution that we've seen in
medical education for that reason.
So I wanna go back to
that concept of comfort
level, especially with AI.
Medicine is a very historically
rigorous evidence-based culture.
AI models, you can't always
tell what's going on inside.
And yet there's so much value in
things like documents summation.
I remember having people talking to
me 10, 15 years ago about the need to
pull in a medical student to do a chart
biopsy on a new patient who came in
and go reading through every previous
node, and I, I, I sort of became very
enamored with that for his chart biopsy.
And I was saying it too often for a
while, AI can do the chart biopsy.
Mm-hmm.
It could probably do the chart
biopsy as well as many medical
students as the provider who's
making a decision about care.
How do you get comfortable?
Yeah, so I wanna approach it
from a couple different angles.
There's a story that I, I routinely
tell that, and there's actually a really
good article by Reinhardt, uh, that was
written in 2020, and it was really about
the stethoscope and how the stethoscope
was ushered in and became something that
physicians had comfort level with, and so
it was invented in the early 18 hundreds.
And at this point, uh, as we think about
its adoption within the United States,
American physicians really had to attend
lectures and, and clinics in Paris to
really learn from some of the fathers
of the stethoscope and relaying it.
René Laënnec was actually
the person who invented it.
And the challenge was, is that it
was limited to very elite physicians
who happened to work in academically
affiliated medical centers.
And so it wasn't every doc who was seeing
every patient that was able to see it.
Adoption was slow, and I think
there was a lot of reasons
why adoption was challenged.
There was a lack of formal education.
You know, there wasn't really a
lot of bedside training that was
available with the stethoscope.
There was a complexity that existed
in the way interpretation happened.
And how to sort of, what do we do with
this atory information that's coming?
There was a hesitancy to create
this barrier between the patient
and a and a clinician by putting
an instrument in between them.
And then there wasn't really a lot of
opportunities for continuing medical
education that physicians could join
into after they've left medical school.
And so a lot of the learning tended to
stop, you know, when they left medical
school and that was, so, it was probably
a little less than a full century
before adoption was so commonplace and
it would be hard, you'd be hard pressed
to find a physician that graduates
from medical school that doesn't
own a stethoscope, hasn't used one.
And granted, there's technology
that's evolved to replace a lot
of the things that we did with
stethoscopes and do so better.
But it's such a central concept
to how we trained as physicians.
And I think we're going through
some of the same challenges with
artificial intelligence right now.
There's a lot of information that's
coming at us, a lot of noise that
exists, a lot of bias, a lot of
reluctance to adopt beause of the fact
that we're fearful that it creates
a, a wedge between us and patients.
I think there's a lot of value to provide
that, that AI provides in doing some of
the things that tend to be very root.
Quite honestly, physicians don't
have the time to deal with.
If you go to an emergency department and
you ask the average doc, how many pages
do they sift through of a person's past
medical history before they abandon and
ship, and then go in and ask the patients,
I think it's, the number is
somewhere around like seven pages.
Right?
It just, there's so much information
that's gathered in that medical chart.
Some, a lot of which is repetitious,
a lot of which may not even be correct
in terms of the way it's captured.
AI is a way of kind of sifting
through that and making it
so that it's streamlined.
There's so much nuance
that isn't captured.
Like I, I used to think about the way
I used to write my clinical notes.
I would go in and have an interview
with a patient and have a, it was a
conversation and then translating that
conversation into something that would
be necessary to, to document code and
bill, I'd lost so much of the nuance that
was so critical for differentiating some
of the gray zones in people's diseases,
and that information was lost forever.
And so things like ambient listening,
while they may be something of
uncomfort in the room at the
point that it's being captured.
Oftentimes provide much more granularity
and much more nuance that's really
important to ultimately making
the right decision for patients,
making the right diagnosis, and
prescribing the right treatment.
I, I do think that there is going to
be an adoption issue and a comfort
level that people are gonna have to get
through, but there's no way that it's
sustainable at this stage of the game.
Where a clinician sees a patient, you
know, and for every hour that they
spend seeing a patient, they document
for another half hour after that.
Right.
That's just not sustainable when
we're, it's sort of seeing the
dwindling numbers of providers and
increased numbers of patients that
are increasingly becoming complex.
In terms of how we adopt it's
really the thoughtful learnings from
technologies like the stethoscope that
we think about how we as a medical
discipline start to adopt technology
in a way that's that's comfortable.
Thinking a a little bit more about some
of the, you know, the natural language
processing element you were just talking
about clinical documentation, do a little
experiment, like if you get a letter back
from a, on a patient you sent out for
a consult, and the first line is, thank
you for this very interesting consult.
What do you read between the lines there?
I've
had that statement represent a
whole bunch of different things.
One thing is, I don't know why you
sent this to us, because you probably
could have figured it out on your own.
You're a primary care doc and you
practiced internal medicine and
you went to residency with me.
I'm calling it interesting, but
it's a little tongue in cheek and
this is probably something you
could have figured out on your own.
I've been in academic medical centers
where there's some sincerity to that.
There's there there's some, it's
a convoluted case that involved
a lot of information gathering.
Perhaps I, as the primary care doc
have gathered some of that information
and synthesized it in a way that I
was able to send it to a specialist
and the specialist pieced it all
together and because of their area of
expertise came to an easier diagnosis.
I've seen both of that, right?
Both of those circumstances where on
one hand it's, hey, I just realized
that the reason why you sent me
this patient is because I'm an ENT
doc and this person had a nose.
And then other times it's, hey,
this is a really complex case,
you synthesized this very nicely
and I got a lot of information.
But I think like I think when
you look at AI from a medical
decisioning standpoint, some of that
information gets glossed over, right?
What's really of essence is, hey, this
is a 53-year-old male with past medical
history, significant for A, B, C.
They're on these medications, these are
their allergies, these are social vices.
They presented to you with
a complaint of X and this is
what you saw on physical exam.
I substantiated by finding this.
I did a couple of extra tests
and in the end I've come to the
conclusion that this is what the
diagnostic, the diagnosis is, right?
It really, I think, a good consult
letter not only starts with those sort
of entry statements and sort of exit
statements, but they really have a lot
of information about the Socratic method
that medicine has practiced in, right?
How we gather information from a
subjective objective, make an assessment,
make a plan for what this patient has.
And that's where I think AI has
become really good at pulling out of
these consult notes to really help
make informed clinical decisions.
And that was the theme that I was hoping
that we could dig in on a little bit
because there is so much context and
things that are written and things
that are not written in these notes.
And you know, I remember working with
people years ago where simply pulling
out someone's smoking status from
a, and this was before that was a
required field as part of meaningful
use, was incredibly different.
Difficult 'cause you only had the free
text node and non-smoker quit last
year is very different than non-smoker.
Which is very different than
12-pack-a-day smoker quit 10 years ago.
And pulling that into a format where you
could make a, actually make a decision
is this patient eligible for a trial?
Are they in a real world evidence cohort?
That's interesting, is very
challenging and I, I think while
we have a lot more coded data now,
we have a lot more complexity that
we need to work through as well.
You know, I think you look at SNOMED
for example, it's become really
expansive and you could really capture
a lot of the pros documentation
in, in, in structured format.
It's still not perfect and
it's not a hundred percent.
And there's, I think the other
thing is that as we think
about why physicians document,
yes, some of it is to create
a historical medical record,
but that really drives care.
But there's a whole lot of other
reasons why they document to
protect themselves medical legally.
They document to establish
patient satisfaction.
They document for the purposes
of building and coding.
And so sometimes it's really
difficult to sift through that.
And I think you can look at the
situation where in a consult letter,
there's an intro sentence and
you can decide, all right, what's
ultimately the meaning of that.
But I think if someone, for example,
if you wanna allude to the fact that
someone's, you believe that someone's
an alcoholic without allowing it
to be a risk factor for you from
a, from a medical legal standpoint,
because you didn't address it.
While you also don't want the
patient to see their record where
you're insinuating that they're an
alcoholic, so that you'll lose trust
with a patient who you're trying to
essentially get to the bottom of things.
It's a really dicey way about how to
appropriately document in a clinical
loop, right, and still capture the nuance.
Still capture, still protect yourself
from a medical legal standpoint and
still establish and continue to establish
that trust with the patient because
they ultimately get to see what's
written in there and dissecting that,
from an, from a an NLP standpoint, that
becomes a bit of a challenge, right?
Because you don't know what
the, what were the motives for
documentation in this particular note?
For companies that are developing, whether
it's ambient documentation systems or
other AI assistive technologies, where
should they be focused if in terms
of creating trust with physicians?
Yeah.
I mean, I, I think as I've educated in,
in informatics and what I think, I think a
lot of the, a lot of the times physicians
are afraid that the, these solutions
are coming to, to are being adopted, not
necessarily to drive the improvement of
the patient experience or to diminish
provider abrasion, rather to make
it so that workflows that are more
administrative in healthcare that
lead to better billing and coding
and reimbursement, things like that
are really the motivating factors.
And while I think we always have to focus
on return on investment when it comes to
a lot of these solutions, some of that
investment is in some of that return
comes in the form of a life saved or
a medication error averted, physicians
went to medical school and clinicians
went to their professional school.
Because they really wanna make a
difference in, in people's lives.
And I think we have to resonate with
them as we have these conversations with
solutions that are meaningful and we have
to see whether or not it truly helps it.
It helps diminish provider abrasion by
implementing some of these solutions.
So we wanna make sure that we're
thoughtful about how, what are we
have clinicians understand what the
motives for implementation are, that
they're truly about helping improve the
physician-patient interaction and they're
helping improve the way care is delivery,
and they're helping to reduce some of
the challenges that we have as physicians
from a rote documentation or some of the
administrative billing issues, et cetera.
That's really what I
think physicians want.
So a few minutes ago you mentioned
SNOMED, and that may not be a term
that all the people listening to
the podcast are familiar with.
So for people who are joining us to learn
a little more about the shape of this
healthcare standards and data world,
can you just give us the two or three
minute tour through modern coding system?
Yeah, so I think a lot of times, you
know, as if we're thinking about the
lay people, there's probably two codes
that they, two types of codes that
they see in their medical billing.
For example, and that's usually the
ICD-10, which is the International
Classification of Diseases in the
CPT, which is a, a list of medical
procedures that are done, or, uh, the
types and intensities of visits that are
captured at at, at the point of care.
Those are largely transacted to be
able to drive thoughtful billing.
But electronic health records have
become so much more complex in
terms of the types of data that
sort of, you know, go into them.
And, you know, there's a, a set
of terminology called LOINC that's
responsible for how we capture things like
blood pressure and how we capture parts of
a complete blood count or a comprehensive
metabolic panel or a blood culture.
There's parts of medications that actually
have a set of terminologies that are
associated with how they're captured.
There's a coding standard that
exists for vaccinations, and so a
lot of our vaccine registries are
set up with that coding standard.
And SNOMED is really a, a sort of a
catchall set of terminology that really
captures not only disease states and
their procedures, but also qualifying
statements that tend to have adjective
and adverb qualities associated with
them so that we can get more nuanced.
Understand that ICD-10 only classifies
things in more broad brushes.
I think you can get more specific
information about diseases if
you start bringing in these other
coding standards and really creating
sort of an amalgam of these coding
standards to help tell a story.
And that's, I think the best way to
represent it is that each of these
coding standards really is, represents a
different part of speech, let's just say
in a sentence so that the full compendium
of the medical information that's captured
in that chart has been codified in a
way that can be transacted by machines.
Well, and we want our audience to be
able to sleep tonight so we are not
gonna talk about NDC codes and how
that works with electronic prescribing.
No, no, we're not.
So pulling all this stuff together,
are there categories of applications
that you think are just more feasible
now, thanks to all this progress,
both in standards adoption and in AI?
Like what are you excited about?
Absolutely.
I think, you know, there's been really an
evolution of a lot of different things,
and I think just even through my, my
career, you know, I can come up with
sort of four quick success stories as
different phases in, in, in my informatics
career that have been really impactful.
You know, I think COVID had a lot
to do with how standard adoption and
electronic data capture, we became
more thoughtful about how to do it.
And so I had the opportunity in one
of my former roles to work for a
company that, um, happened to work in
a state at the public health level.
One of the challenges that existed
from an interoperability standpoint
is that there were over a hundred
different ways of capturing
a simple concept like COVID-19 PCR test
and 40 different ways of representing that
patient had a result that was negative.
And so if you look at a hundred
different ways of capturing the test
and 40 different ways of capturing
its negative value, there's 6,000
different permutations that could
exist of how that data's captured.
And so you could see where if you create
data standardization, it really has a huge
ROI impact on public health initiatives.
And again, this was only in one state.
Imagine what this is across, you
know, this is 40 different labs.
Imagine what this is across 400
different labs in 10 states.
Right?
So this, this is a, a real problem
that I think technology was aimed
at solving and did a great job.
I worked with a group of students
addressing how to take medication
instructions and parse and parse
them using not only medication
normalization or, or data
normalization, but then deploying
novel solutions like NLP and Gen AI.
To really get better F1 scores for some of
these solutions, and, you know, the amount
of medication instructions that could
be parsed once normalization is added
to to, to these NLP Gen AI solutions,
uh, makes it so that the capture of
the information is almost near perfect.
So that's, it's speaks to the fact that
we can really reduce medical errors,
which are substantial impact in, into
why people stay in hospitals longer,
and a significant cause of morbidity
and mortality in amongst patients.
And then in sort of my most recent role,
it's really identifying individuals
and pulling together some of this
multi-source and multi-format data,
really pulling in a combination of
social patient reported outcomes
data, pulling in a lot of the medical
data from electronic health records.
A lot of the billing data and sometimes
the incongruence between what's
captured in a chart and what's what's
billed is actually very insightful.
That sort of absence of congruence
is actually very helpful in how
you build machine learning models.
And so we looked at
individuals primarily older age
with clear modifiable risk factors
like things like polypharmacy or
the way their homes were set up
that were at risk for falls and
actually started targeting thoughtful
interventions to prevent those falls.
And this is really showing that having
a combination of data standardization
as the foundation for more advanced
analytics, predictive modeling and AI
is really important to how we make the
job of treating patients and doing so
in a way that's contextually appropriate
and thoughtful and diminishing in the
provider's burden is really very valuable.
So I think those are really four
good examples of how automation
clinical decision support, and sort
of advanced analytics can be used to
help drive healthcare improvement.
So
actually going back to SNOMED.
Just listening to this conversation
as we've been having it, there's
always been an adoption barrier there.
It's obviously a lot of work.
It's a lot more work to enter structured
information and you've gotta create
an incredible user experience to
be able to do that versus being
able to write a narrative note.
With these a newer AI tools and summation
have we been solving the wrong problem
in terms of designing code systems?
Early on, I think the purpose of
coding systems was really automate
the way hospitals worked, automate
the communication between different
areas of the hospital, drive billing.
I even think, by the way, I think that's
a very insightful, insightful question.
I don't think it's even
about coding systems.
I even think that it's about the
reason why the electronic health
record evolved the way it did.
It wasn't.
I, I don't think it was created with
a thought in mind that this is the
best way to capture data, to create a
chart, a living chart for a patient.
It was really about how do we
create something that lends itself
to billing and coding, right?
And not necessarily how we
create something that tells this
person's full medical story.
And so I think the way coding standards
did evolve, or at least the way they were
utilized, was not necessarily for the
primary intent of patient care delivery.
I think we've done a good job of
starting to pivot and really being more
thoughtful about how we use these tools to
identify opportunities and gaps in care.
But I think we've been reactive in the,
in the way we've gone about it, because
again, they weren't set up in a way to
drive true chronicling of medical care.
They were invented for other
purposes, billing, coding,
medical, legal, et cetera.
And I do think that the technological
evolution that we're seeing now
probably is more thoughtful.
I think the role of the physician
and the clinician informaticist
has made it so that we're being
more protective of our turf.
We're not a technology, we're
not a technology person first.
We're a clinician first, right?
And we're building thoughtful technology
solutions that help augment the way we
can deliver better care for patients.
So in a way we're, we're really
almost going back to the beginning.
People like Octo Barnett and Larry
Weed, who were right at the beginning
of this electronic record keeping
revolution back in the sixties and
seventies and had a very clinical,
very patient oriented historian
approach to designing these tools.
And then the process automation
juggernaut took over from that.
I think we, we deviated a little bit
from that purity of intent early on,
and I think that by having clinicians
involved in the process now, where I
think we're getting back to it for sure.
Continuing on on this theme of clinical
adoption, have you seen any organizations
that you've felt have done a, a really
good job and of thinking about the ROI
on some of these clinical informatics
programs and interviewing the work of
their CMIOs into their strategic plans.
Um, I think that there's a
lot of very forward thinking.
I think there's forward thinking hospitals
that have done really good work around
how are we incorporating some of these
solutions in a way that's not just
about checking a box and really bringing
in the CMIO decision making, um, into
the whole, into the whole process?
I think that there's a couple of
very thoughtful states that really
accelerated the sort of modernization
strategies during the COVID pandemic
and did so in a way that was thoughtful.
I think you're starting to see a lot
of tech enabled organizations that kind
of have come out that are more maybe
concierge based or even concierge light.
They don't demand sort of a lot
of, a lot of membership dues for
patients, but ultimately really
deliver hugely tech-enabled solutions.
I think that there's, I, you know,
look, I, one of the, one of the
indictments that I have of meaningful
use is that I think that there wasn't
necessarily the right carrot and there
wasn't necessarily the right stick.
Right?
It became a really big challenge
to get providers to engage because
of the fact that it was such a
laborious process to get people on
board, and then the penalties for
non-engagement were actually piddly,
especially if you didn't necessarily
have a big Medicare or Medicaid
population in your patient portfolio.
And so I think that people adopted
and there was a lot of checking
boxes to say that they adopted.
A lot of the initial meaningful use
requirements were self-report, and I think
when you have that kind of self-report
and you don't have the right incentives
to get people to do it or disincentives to
prevent people from not doing it, I don't
think that there's thoughtful adoption.
One of the challenges that's existed
is, is that while the capacity exists
to allow people to do that the education
doesn't necessarily exist, and I
think there are thoughtful hospital
systems, thoughtful payers, thoughtful
providers that are really making
patient education and patient navigation
about how to use those solutions
a central part, you need to get, I mean,
I think the two biggest adopters that need
to drive this are your medical staff and
your clinical staff and hospitals, and
then you gotta get patients on board with
understanding about how to do to do this.
It's such a complex care delivery model
that unless they're fully bought in,
you could have patient right of access.
No one's accessing it.
And so I think, I think that's really
what's required to have success.
It's really the buy-in of both
the clinician staff as well as the
patients, regardless of whether
it's a payer provider, hospital
system, a concierge practice, a
tech enabled practice, et cetera.
Those are the things that
are absolutely required.
I'm
reminded of a conversation from 15,
20 years ago with the uh, CIO of a
hospital system that had one of the
first patient portals and he told me two
things that people use it for refills and
appointments, and it's paid for out of the
marketing budget, not out of the clinical
budget, not out of the core IT budget.
And really they saw it as a tool for
retaining their patient population.
What are some of the levers that, like
that convenience of being able to make
an appointment or send a message to your
clinician that will help drive patient
adoption of these new care models.
So I, I think a lot of
it is really access.
You know, you look at things like
mental health, there's some states,
and we're not talking about states
that are necessarily significantly poor
states, and we're talking about affluent
states that still have challenge with
people being able to access crisis
care from a mental health standpoint.
There, there's such an issue
that in one of my previous
roles, I actually did a study on
the incidents of somatization, so
people presenting with physical
complaints for psychiatric illness has
gone up because of the fact that they
oftentimes can access mental health
or can't access the appropriate type
of mental health, or there's social
stigma as associated with mental health.
Or you even look at mental health
providers just being able to find a
psychiatrist or a psychologist or a
social worker that's in your network
that can see you any, anything
sooner than two months from now
has been a significant issue.
And you know, you can see where solutions
like telemedicine, you can see where the
ability to ask patients' questions in a
way that's re recursive allows people,
allows it to serve as a triage system
that can identify those people that
truly need to be seen much more rapidly
and those that perhaps can wait.
You know, one of the stories I tell is
about a family member of mine that had
respiratory complaints and ended up
getting admitted and nearly intubated
because they went to an emergency
department at the same hospital three
separate times complaining of shortness
of breath and on three separate times,
got antibiotics as the prime modality of
treatment for a diagnosis of pneumonia.
And it turns out that this person never
had pneumonia and if they just looked
into their medical records and accessed
them in a way that was easier than
having to look at pieces of paper or
duplicate charts, you know, where there
was cut and paste, where you had an
active comprehensive problem list that
was informatically coded into the chart
you could really pull together information
and synthesize that information in a
way to come up with a very thoughtful
differential diagnosis about what
was going on with this patient.
It turns out that this
patient didn't have pneumonia.
They had pneumonitis, and if they just
went back to their gastroenterology
records and they went back to their
neurology records, they would see
that in addition to respiratory
complaints, they had GI complaints
and they had neurologic complaints.
And it turns out that this was the
first set of symptoms that this
person was declaring themselves as
having a rheumatologic illness with.
So I, I, I think the ability to put
information at the hands of clinicians in
a thoughtful way, not putting the burden
on the patients to tell their story every
time they go into the emergency department
or every time they see a new specialist.
I mean, that's a huge absolution
for patients of having to do that
every time they, they seek care.
And think about it, that the, the more
complex you are as a patient, the more
you're gonna likely have to become an
expert in your own diagnosis in order
to basically transact your care because
your medical chart has grown to the
point that there's thousands, tens of
thousands of pages, and you need to be
able to synthesize and summarize that
so you know that the medications that
you've been given have worked, and these
are the side effect profiles that you've
had, and this is the medication that
didn't work, and this is what ended up
happening when you took this medication.
Like all of that information should
be in a way that's accessible so that
it lends itself towards appropriate
clinical decisioning, be it automation
by machines or improved rate of,
of speed by a clinician who's able
to look at that information on one
screen and say, aha, this is what's
going on with this individual.
It looks like pneumonitis
because they have GI issues and
they have neurologic issues.
So that's a good segue into talking a
little bit more about equity and access.
So, how both with improvements that
we have made around standardization
of healthcare data and of course
these newer AI enabled technologies,
how do you see that helping bridge
some of the gaps in healthcare access?
Like we talked about it a little bit
in the mental health context, but
I'm sure there's quite a few others.
Yeah, I mean, I, I think it comes
with some pitfalls that I think
we need to be concerned about.
I think one of the things that I did
in a study at one of my, recent job
was I, I looked at data quality as it
varied by demographic features, how as
it varied by socioeconomic status, race,
ethnicity, languages spoken, et cetera.
We have a significant challenge when
it comes to data equity, um, in the
United States, and not everybody
has their data captured at the point
of care in an equivalent fashion.
And a lot of this has to do with ways
that people utilize the healthcare system.
I think in areas where
there is provider mistrust
I think the emergency department
tends to be a big source.
I think you fail to create a longitudinal
record that tells a full story.
Even for chronic illness it tends
to be an episodic care delivery
model for these chronic illnesses.
And so a lot of times you're not really
getting a robust data capture, and
that's, it's one of the many reasons
why you see such social disparities
in the treatment of chronic illness
is that some people receive their
chronic illness care as a series of
independent acute illness representations.
They go to the emergency department
when they feel dizzy because their
blood sugars are running 400.
Instead of having proactive care
that drives, that helps them so
that their sugars are never 400.
So we need to identify that there's
a substantial data equity piece.
When we're looking at build, you know,
machine learning models, one of the
challenges that exist there is that,
you know, your features may be the
same for two diverse populations, but
the way diagnoses are captured may
be vastly different to the point that
we may underestimate the prevalence
in a population of a certain disease
entity, or because of social stigma, it
may actually present with a different
ICD-10 code than depression, right?
They may have a series of somatic
presentations of, uh, mental illness
that we should have built-in ways in
machine learning models that identify,
hey, this person's now been seen for
palpitations, headache, abdominal pain,
alternating diarrhea and constipation.
Perhaps there's a mental health
issue that we need to address.
Perhaps that should be built into the way
that we think about how this population
in this geography or in this community
tends to represent the way it, you
know, mental health gets represented.
And so I think the data
equity piece is a big one.
We need to understand that.
We need to make sure that we're
considering the cultural context, we're
considering broadened data sources.
You know, not only some of the medical
data, but also some of the social data is
really important for certain populations.
Um, we wanna do even stratified
sampling within some of those
populations to make sure that we're
doing things in a way that's fair
from a, an algorithmic standpoint.
And at times where there's
data that's missing, we need to
understand disease prevalence in
certain populations and even do data
augmentation until we get to a point
where data capture is seen as more
equitable for certain communities.
So you started your career on
the practice side and then made
transition into clinical informatics,
but never really stepped away
from the practice element either.
So for other physicians who are interested
in getting more deeply involved with
building digital healthcare technology
and wanna make that transition either
partially or completely into the
healthcare technology world, what
would you suggest they keep in mind?
Medical education's evolved and has
needed to evolve because of how much
technology that exists in healthcare.
You know, I think that different
schools have evolved in different cases.
Um, I would say that those students of
medicine that are kind of going through
the, the, the programming now take the
opportunity to learn with people that
are doing different things in healthcare.
And they'll feel that because of the
fact that you went to medical school,
that there's only one traditional way of
practicing medicine and delivering, you
know, value from a healthcare standpoint.
I think there's a lot that
needs to be fixed in healthcare.
Um, there's a lot of policy
issues that that PD addressed.
Um, there's a lot of the different
ways we transac healthcare.
There's a lot of gaps when it comes
to care and ation, and it's one of the
things that I was kind of mentioning
is previously, uh, patients don't know
how you use healthcare in the United
States, like I had the opportunity to
work in an urgent care center like it,
it was a mixture of people that truly
needed to be there because they had
something that acquired urgent care.
And then I would say the other half of
the people were people that probably
either to be an emergency department and
passed six of them along the way, uh, in
order to get to this urgent care center.
And there were, there were people
that would come in and they had
six months worth of the complaints
that probably could be better dealt
with with a primary care doctor.
People don't know how to
use the healthcare system.
I think that we as clinicians can
be empowered as of getting people
to, you know, use it in, in a way
that's contextually appropriate.
And then obviously, you know, in many
of the conversations that we had, you've
had, there's a gap in, in, in terms
of the resources that are necessary
to provide care to whatever complex
and ever aging, you know, population.
And so technology's gonna have
be the way to, to mitigate that.
And so really identifying that you can
provide value with your clinical know-how,
in a way that's non-traditional is really
very, very valuable for, for, you know,
clinicians of medicine to understand.
And then the opposite of that, for
all the non-clinicians in the audience
who want to get involved in healthcare
technology and improving the way that
we deliver care, whether it's in,
in this country or globally, where
should they focus, you know, as they
build their foundational knowledge of
clinical informatics and
interoperability in healthcare, AI?
Some of the more successful companies,
um, when, when it comes to tech, new
technology companies, some of the more
successful companies in healthcare
are first and foremost the health
solutions company, and they have the,
the, the key clinical pieces in place.
Healthcare is nuanced, right?
It's, it's not like there's a binary
outcome for every compilation of tests.
Not every test combination
adds up to the same diagnosis.
There's a lot of many to one, one to many
relationships that exist in healthcare.
There's a lot of interrelationships
that exist and there's no one
way that a diagnosis presents.
There's a lot of nuance
to how they presents.
There's not a one size fits all treatment
when it comes to, you know, hypertension.
We often look at people with hypertension
who have other comorbid illnesses, may
respond to different anti-hypertensive
medications, may actually have those
anti-hypertensive medications treat
some of their other comorbid illnesses
if the right medication is selected.
So as people that are studying technology,
studying data science, studying machine
learning, AI, et cetera, understand
that medicine is binary, it's nuanced.
Um, there's a lot of drivers of medicine
that are not just simply, purely based
on, you know, how someone presents what
diagnosis they have of what treatment
they receive, but there's a whole lot of
interplay from social factors, et cetera.
Having clinicians on board to learn,
knowing, knowing how to talk the talk and
walk the walk of, of, of the clinician
is important to adoption, right?
Because there are stakeholders that
oftentimes may get overlooked and these,
these technology solutions get implemented
in these, you know, hospital systems and
provider offices, et cetera, and they're
the ones that could be part of your
greatest success on a go forward basis.
So, you know, I would say understand
the limitations of AI, the biases
that exist in AI for something as
convoluted as as the medical sciences
and learn from those medical scientists.
The healthiest organizations I've been
a part of, I've taught as much medicine
as I've, as I've learned data science.
I've taught as much medicine as
I've learned standards, right?
Um, and I think that that bi-directional
exchange of information really makes
growing organizations and really
deliver, delivers the best ROIs
So, uh, if our listeners could take, uh,
one thing away from this conversation
that we've had over the last hour or
so, what would you like that to be?
So, I think the time is now.
Setting aside adoption, setting aside,
you know, some of the challenges that
exist from the bias standpoint, setting
aside some of the, uh, challenges that
exists from patients and providers
and technology solutions, et cetera.
We need to reform the way healthcare
is delivered in a way that's equitable,
in a way that's thoughtful and
a way makes the right diagnosis,
prescribes the right treatment.
At the end of the day, technology
enablement are really related.
Um, and having the right organizational
mixture of clinicians and technologists
at a company is vital key to success,
not only of that company, but to the
entire healthcare delivery model.
Thank you very much.
Dr. Pinho.
Thank you so much for being
with us this afternoon.
I think that this conversation is
gonna provide a lot of great context
for listeners as they continue
their own journeys in health IT..
This has been Hard Problems, Smart
Solutions, the Newfire podcast.
Thanks for listening and come back
next time when we'll be diving
into interoperability strategy and
digital health product management
with podcaster Omar Muza.
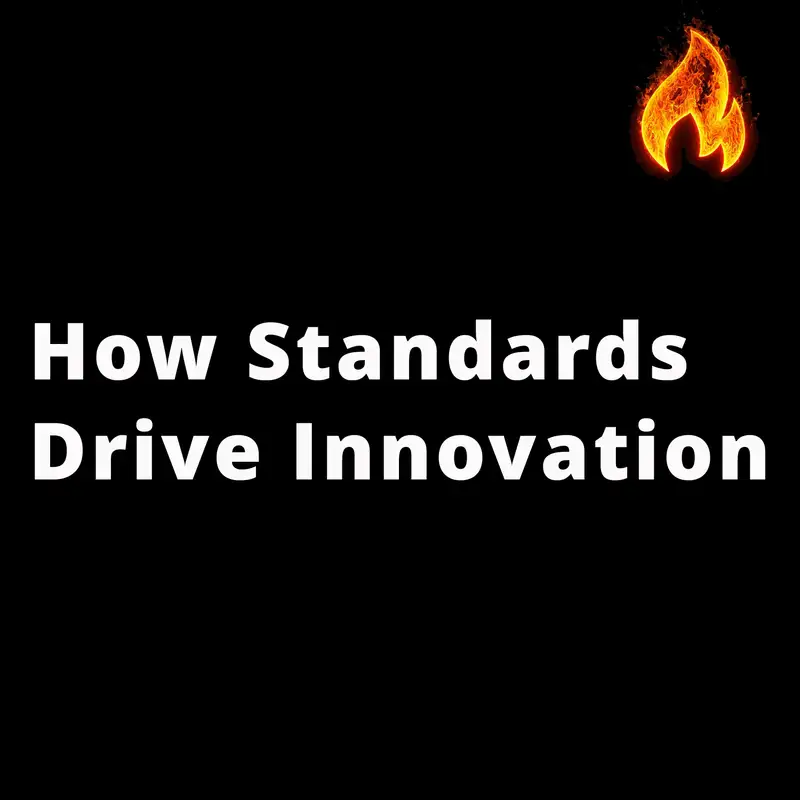